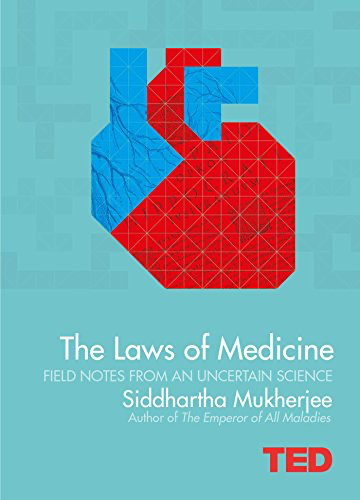
Finished on 30/1/20 in Sunshine Coast, Australia.
"I had never expected medicine to be such a lawless, uncertain world. I wondered if the compulsive naming of parts, diseases, and chemical reactions - frenulum, otitis, glycolysis - was a mechanism invented by doctors to defend themselves against a largely unknowable sphere of knowledge. The profusion of facts obscured a deeper and more significant problem: the reconciliation between knowledge (certain, fixed, perfect, concrete) and clinical wisdom (uncertain, fluid, imperfect, abstract)."
"Every diagnostic challenge in medicine can be imagined as a probability game. This is how you play the game: you assign a probability that a patient's symptoms can be explained by some pathological dysfunction - heart failure, say, or rheumatoid arthritis - and then you summon evidence to increase or decrease the probability. Every scrap of evidence - a patient's medical history, a doctor's instincts, findings from a physical examination, past experiences, rumors, hunches, behaviors, gossip - raises or lowers the probability. Once the probability tips over a certain point, you order a confirmatory test - and then you read the test in the context of the prior probability."
"It is here that an insight enters our discussion - and it might sound peculiar at first: a test can only be interpreted sanely in the context of prior probabilities.
It seems like a rule taken from a Groucho Marx handbook: you need to have a glimpse of an answer before you have the glimpse of the answer (nor, for that matter, should you seek to become a member of a club that will accept you as a member).
To understand the logic behind this paradox, we need to understand that every test in medicine - any test in any field, for that matter - has a false-positive and false-negative rate. In a false positive, a test is positive even when the patient does not have the disease or abnormality (the HIV test reads positive, but you don't have the virus). In a false negative, a patient tests negative, but actually has the abnormality being screened for (you are infected, but the test is negative). The point is this: if patients are screened without any prior knowledge about their risks, then the false-positive or false negative rates can confound any attempt at diagnosis. Consider the following scenario. Suppose the HIV test has a false-positive rate of 1 in 1,000 - i.e., one of out every thousand patients tests positive, even though the patient carries no infection (the actual false-positive rate has decreased since my time as an intern, but remains in this range). And suppose, further, we deploy this test in a population of patients where the prevalence of HIV infection is also 1 in 1,000. To a close approximation, for every infected patient who tests positive, there will also be one uninfected person who will also test positive. For every test that comes back positive, in short, there is only a 50 percent chance that the patient is actually positive. Such a test, we'd all agree, is not particularly useful: it only works half the time. The “more thoughtful internist" in our original scenario gains very little by ordering an HIV test on a man with no risk factors: if the test comes back positive, it is more likely that the test is false, rather than the infection is real. If the false-positive rate rises to 1 percent and the prevalence falls to 0.05 percent - both realistic numbers - then the chance of a positive test's being real falls to an abysmal 5 percent. The test is now wrong 95 percent of the time.
In contrast, watch what happens if the same population is preselected, based on risk behaviors or exposures. Suppose our preselection strategy is so accurate that we can stratify patients as "high risk" before the test. Now, the up-front prevalence of infection climbs to 19 in 100, and the situation changes dramatically. For every twenty positive tests, only one is a false positive, and nineteen are true positives - an accuracy rate of 95 percent. It seems like a trick pulled out of a magician's hat: by merely changing the structure of the tested population, the same test is transformed from perfectly useless to perfectly useful. You need a strong piece of "prior knowledge”- I've loosely called it an intuition - to overcome the weakness of a test."